Convergence AI Releases WebGames: A Comprehensive Benchmark Suite Designed to Evaluate General-Purpose Web-Browsing AI Agents
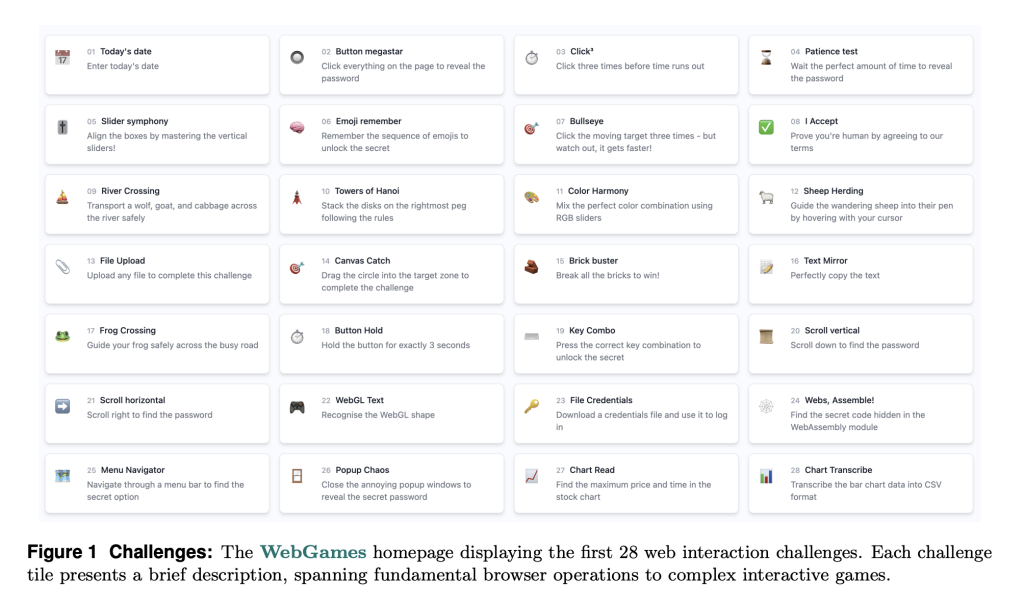
AI agents are becoming more advanced and capable of handling complex tasks across different platforms. Websites and desktop applications are intended for human use, which demands knowledge of visual arrangements, interactive components, and time-based behavior. Handling such systems requires monitoring user actions, from clicks to sophisticated drag-and-drop actions. Such challenges are difficult for AI to handle and cannot compete with human capability regarding web tasks. A broader evaluation system is necessary to measure and improve AI agents for web browsing.
Existing benchmarks evaluate AI performance in specific web tasks like online shopping and flight booking but fail to capture the complexity of modern web interactions. Models such as GPT-4o, Claude Computer-Use, Gemini-1.5-Pro, and Qwen2-VL struggle with navigation and task execution. Initially based on reinforcement learning, traditional evaluation frameworks expanded to web tasks but remained limited to short-context scenarios, leading to quick saturation and incomplete assessments. Modern web interaction requires advanced skills like tool usage, planning, and environmental reasoning, which are not fully tested. While multi-agent interactions are gaining attention, current methods do not effectively evaluate collaboration and competition between AI systems.
To address the limitations of current AI benchmarks in web interaction, researchers from Convergence Labs Ltd. and Clusterfudge Ltd. proposed WebGames, a framework designed to evaluate web-browsing AI agents through over 50 interactive challenges. These challenges include basic browser usage, complex input management, mental thinking, workflow automation, and interactive amusement. Compared to the prior benchmarks, WebGames intends to measure correctly by separating interaction skills and providing tested AI with control. Its client-side design prevents dependencies on external resources, providing uniform and reproducible tests.
WebGames is modular in design. It specifies problems in a standardized JSONL format for effortless integration with automated test frameworks and extension with additional tasks. All problems follow a deterministic verification structure that ensures task verifiability when it is done. The structure examines AI performance in a systematic way through web interactions, quantifying navigation, decision-making, and adaptability ability in dynamic environments.

Researchers evaluated leading vision-language foundation models, including GPT-4o, Claude Computer-Use (Sonnet 3.5), Gemini-1.5-Pro, Qwen2-VL, and a Proxy assistant, using WebGames to assess their web interaction capabilities. Since most models were not designed for web interactions, they required scaffolding through a Chromium browser using Playwright. Except for Claude, the models lacked sufficient GUI grounding to determine exact pixel locations, so a Set-of-Marks (SoMs) approach was used to highlight relevant elements. The models operated within a partially observed Markov decision process (POMDP), receiving JPEG screenshots and text-based SoM elements while executing tool-based actions through a ReAct-style prompting method. The evaluation showed that Claude scored lower than GPT-4 despite having more precise web control, likely due to Anthropic’s training restrictions preventing actions resembling human behavior. Human participants from Prolific completed tasks easily, averaging 80 minutes and earning £18, with some achieving 100% scores. The findings revealed a wide capability gap between human and AI abilities, much like the ARC challenge, with some activities such as “Slider Symphony” demanding exacting drag-and-drop capabilities that proved difficult for models to accomplish, revealing limitations in AI abilities to interact on real-world websites.

In summary, the proposed benchmark found a significant gap in human vs. AI performance for web interaction tasks. The best-performing AI model, GPT-4o, only achieved 41.2% success, whereas humans achieved 95.7%. The results revealed that current AI systems struggle with intuitive web interaction, and constraints on models like Claude Computer-Use still impede the task’s success. This approach can be used as a reference point for further research, with improvements in AI flexibility, reasoning, and web interaction efficiency being directed.
Check out the Paper and GitHub Page. All credit for this research goes to the researchers of this project. Also, feel free to follow us on Twitter and don’t forget to join our 80k+ ML SubReddit.
🚨 Recommended Read- LG AI Research Releases NEXUS: An Advanced System Integrating Agent AI System and Data Compliance Standards to Address Legal Concerns in AI Datasets
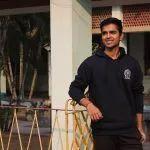
Divyesh is a consulting intern at Marktechpost. He is pursuing a BTech in Agricultural and Food Engineering from the Indian Institute of Technology, Kharagpur. He is a Data Science and Machine learning enthusiast who wants to integrate these leading technologies into the agricultural domain and solve challenges.