Shanghai AI Lab Releases OREAL-7B and OREAL-32B: Advancing Mathematical Reasoning with Outcome Reward-Based Reinforcement Learning
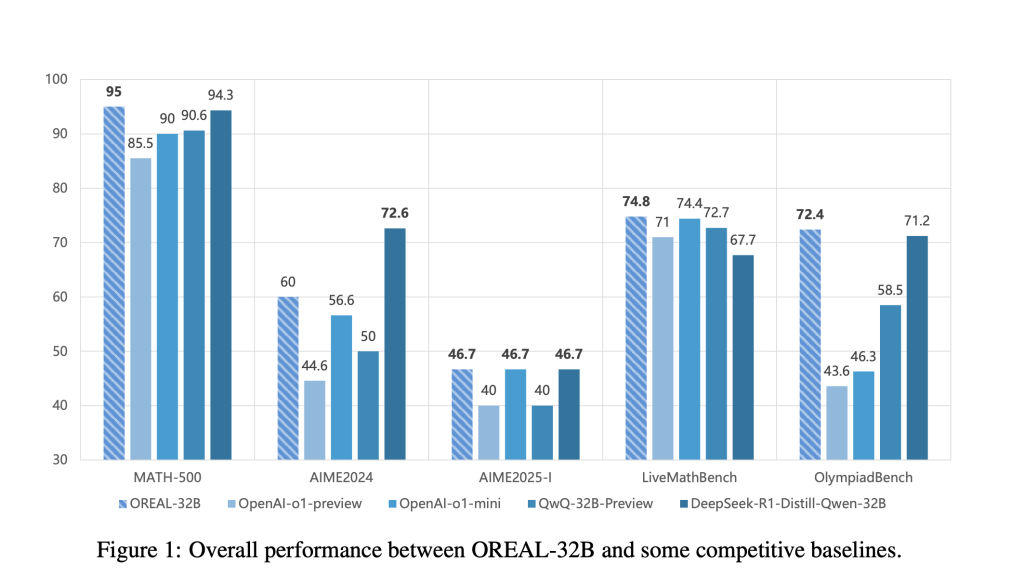
Mathematical reasoning remains a difficult area for artificial intelligence (AI) due to the complexity of problem-solving and the need for structured, logical thinking. While large language models (LLMs) have made significant progress, they often struggle with tasks that require multi-step reasoning. Reinforcement learning (RL) has shown promise in improving these capabilities, yet traditional methods face challenges when rewards are sparse and binary, providing little feedback beyond a correct or incorrect answer.
Shanghai AI Laboratory has developed Outcome REwArd-based reinforcement Learning (OREAL), a series of mathematical reasoning models available as OREAL-7B and OREAL-32B. This framework is designed for situations where only binary rewards—correct or incorrect—are available. Unlike conventional RL approaches that rely on dense feedback, OREAL uses Best-of-N (BoN) sampling for behavior cloning and reshapes negative rewards to maintain gradient consistency.
OREAL-7B and OREAL-32B demonstrate that smaller models can perform competitively with significantly larger models. OREAL-7B achieves a 94.0% pass@1 score on the MATH-500 benchmark, a result comparable to previous 32B models, while OREAL-32B reaches 95.0% pass@1, surpassing previous models trained through distillation.
Technical Insights and Advantages
The OREAL framework introduces several key techniques to improve mathematical reasoning:
Best-of-N Sampling for Behavior Cloning: BoN sampling helps select optimal positive reasoning trajectories, allowing the model to learn from well-formed solutions.
Reward Reshaping for Negative Samples: By adjusting negative rewards, the framework ensures gradient consistency between correct and incorrect samples, refining model optimization.
Token-Level Reward Model for Chain-of-Thought Reasoning: Mathematical reasoning often involves long sequences of logical steps. OREAL assigns importance weights to key reasoning tokens, addressing the challenge of sparse binary feedback.
On-Policy Reinforcement Learning: The model dynamically refines itself based on sampled queries, improving training efficiency and adaptability.
These techniques enable more stable training and better performance in long-sequence reasoning tasks, making reinforcement learning a viable alternative to traditional distillation approaches.
Performance and Evaluation
OREAL models have been tested across several benchmarks:
MATH-500 Benchmark:
OREAL-7B achieves 94.0% pass@1, a performance level previously seen only in 32B models.
OREAL-32B achieves 95.0% pass@1, setting a new standard in mathematical reasoning.
AIME2024 and OlympiadBench:
OREAL models outperform multiple baselines, showing strong generalization across problem types.
Comparison with OpenAI o-series and DeepSeek Models:
OREAL-32B surpasses DeepSeek-R1-Distill-Qwen-32B and OpenAI-o1-preview, demonstrating effective training strategies.
OREAL-7B achieves results on par with QwQ-32B-Preview and OpenAI-o1-mini, highlighting the impact of its reinforcement learning approach.

Conclusion
Shanghai AI Lab’s OREAL-7B and OREAL-32B models offer a refined approach to reinforcement learning in mathematical reasoning. By addressing the challenge of sparse binary rewards through Best-of-N sampling, reward shaping, and token-level importance weighting, these models achieve competitive performance even at smaller scales. The OREAL framework provides valuable insights into how reinforcement learning can be optimized for complex reasoning tasks, suggesting new directions for improving AI’s problem-solving capabilities in structured domains.
Check out the Paper, OREAL-7B and OREAL-32B. All credit for this research goes to the researchers of this project. Also, don’t forget to follow us on Twitter and join our Telegram Channel and LinkedIn Group. Don’t Forget to join our 75k+ ML SubReddit.
🚨 Recommended Open-Source AI Platform: ‘IntellAgent is a An Open-Source Multi-Agent Framework to Evaluate Complex Conversational AI System’ (Promoted)
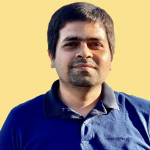
Asif Razzaq is the CEO of Marktechpost Media Inc.. As a visionary entrepreneur and engineer, Asif is committed to harnessing the potential of Artificial Intelligence for social good. His most recent endeavor is the launch of an Artificial Intelligence Media Platform, Marktechpost, which stands out for its in-depth coverage of machine learning and deep learning news that is both technically sound and easily understandable by a wide audience. The platform boasts of over 2 million monthly views, illustrating its popularity among audiences.